Comparison and modification of survival predicting system for breast cancer patients with bone metastases
Introduction
Breast cancer is the most common cancer type that contributes to about one-third of all female cancer cases which is predicted to have around 0.276 million cases in 2020 in the U.S. and 0.279 million cases in China in 2014 (1,2). With the improvement of treatment regimens in breast cancer, especially hormone therapies and targeted therapies, the survival of patients with breast cancer bone metastases (BCBM) has significantly increased as compared to lung and gastrointestinal cancers (1,2). As a result, an increasing number of BCBM patients are suffering from skeletal-related events (3).
Destruction of bone by metastatic disease reduces its loadbearing capabilities and results initially in microfractures. Microfractures can cause pain and eventually may lead to a complete fracture of the bone. Most bone lesions develop symptoms such as consistent pain and impaired function (3,4). Surgery is most commonly needed for mechanical complications, such as a complete or pending fracture or intractable pain (4,5). The goals of surgery are to relieve the pain, restore structural stability and function, improve life quality, and eventually benefit the patient without increasing the risk of complications. Surgical treatment for metastatic disease is palliative and not curative. In oncologic orthopedics, surgical treatment choice varies from less-invasive stabilization to tumor resection and prosthetic reconstruction procedures. The method of choice depends on patient survival and the site of metastasis. Expected survival is the most crucial factor in determining the treatment modality. An accurate survival estimation will help decide the proper surgical plan and treatment regimen, thereby preventing overtreatment and undertreatment.
Several studies had tried to assess the survival time for patients with bone metastases previously, including Katagiri et al. (referred to as the Revised Katagiri model) (6), Janssen et al. (referred to as the Janssen nomogram model) (7), Willeumier et al. (referred to as the OPTI model) (8), Ratasvuori et al. (referred to as the Scandinavian Sarcoma Group SSG model) (9), Forsberg et al. (referred to as the PathFx model) (10), Sørensen et al. (referred to as the SPRING model) (11), and van der Linden et al. (referred to as the SORG model) (12). However, there is no consensus regarding which prognostic model is the most accurate. These models provide inconsistent survival predictions for a given patient at various time points. Lack of external validation could be one of the reasons, especially non-western patient cohorts. Besides, since cancer biology plays a dominant role in patient survival, increasing evidence suggests that tumor type-specific prognostic parameters could contribute to prognostic models’ accuracy (13,14).
Therefore, the present study aims to compare the most prevalent scoring systems available to determine BCBM patients’ survival at various time points. Besides, we use univariate and multivariate Cox regression to identify independent prognostic factors. A BCBM-specific scoring system was further generated with cancer-specific prognostic variables to increase prediction accuracy and guide surgical intervention.
We present the following article in accordance with the STROBE reporting checklist (available at http://dx.doi.org/10.21037/aoj-20-120).
Methods
Study design and subject inclusion
This research was conducted in accordance with the Declaration of Helsinki (as revised in 2013). Ethics approval was obtained from the ethics committee of Peking University People’s Hospital. Informed consent was not required for this retrospective, non-interventional study, which was considered minimal risk. Patients who underwent surgery for breast cancer metastatic bone tumors at a single institution between 2010 and 2018 were investigated. The inclusion criteria were (I) patient age greater than 18 at the time of surgery; (II) complete and detailed electronic medical records with clinical presentation, imaging, histological and operative information available; (III) breast cancer patient who underwent surgical treatment of a metastatic bone lesion; (IV) pathologic confirmation of primary tumor histology; (V) known survival or most recent follow-up. Patients for whom the date of death was missing due to loss of follow-up were censored at the last time they were known to be alive.
Prognostic models
All patients were assessed using the seven scoring systems most represented in the literature. These scoring systems were calculated based on retrospective data before the time of surgery (6-12). Only objective inputs were utilized. The PathFx model has optional subjective variables that were omitted from the model for this analysis (10). Patient data were complete for all prognostic models at the time of operative management. A survival probability was calculated for each patient with respect to the given scoring system.
Statistical analysis
Primary demographic data were summarized as categorical variables and mean with range, standard error (SE), or percentage. To assess which variables were marginally significantly associated with survival, we performed univariate Cox regression analysis on all variables collected. Categorical variables were established as body mass index (BMI) less than 20 kg/m2, age greater than 70 years old, presence of visceral metastases, presence of multiple skeletal metastases, presence of pathological fractures, history of systemic chemotherapy, radiation therapy, hormonal therapy, and bisphosphate therapy. The Karnofsky Performance Score (KPS) greater than 70 versus less than 50 and the intermediated. The Eastern Cooperative Oncology Group (ECOG) performance status equal and less than 2 versus higher than 2. Preoperative lactate dehydrogenase (LDH) greater than 250 U/L, albumin less than 35 g/L, total bilirubin greater than 23 µmol/L, platelets less than <80×109/L, hemoglobin less than 8.5 g/dL and serum calcium greater than 2.6 mmol/L. Molecular type classification of breast cancer, luminal A versus luminal B, HER-2 overexpression, triple-negative and undetermined, breast cancer receptor status of hormone-dependent versus hormone-resistant. A multivariate Cox proportional hazards model was constructed using all the marginally significant variables (P<0.10). Subsequently, covariates that were not independently associated with survival (P<0.05) or violated the proportional hazards assumption were removed in a stepwise manner. All patients were categorized into molecular subtype groups defined by Perou et al. and Sørlie et al. (15,16). The final variables with an independent and statistically significant association with patient survival were retained and reported. The predictive abilities of prognostic scoring algorithms were tested using receiver operating characteristic (ROC) analysis at 1-, 3-, 6-, 12-, 18- and 24-month post-surgery time points using the calculated area under the curve (AUC) and 95% confidence intervals (CIs) for each model. An AUC of 1.0 indicates perfect accuracy, whereas an AUC of 0.5 indicates no relationship or predictive accuracy. An AUC cut-off was set at 0.70 for a scoring system to be considered to have sufficient predictive accuracy (17-19). AUC values were compared with Delong’s test (20). The level of significance was set at P<0.05. The Brier score measures a probabilistic model’s accuracy summing the difference between expected survival and actual survival period. The scores vary between 0 and 1, with a lower score indicating better predictions for the model. All statistical analyses were performed using R software, version 3.5.3. The ROC curve and the AUC were evaluated using the “survivalROC” package, while Kaplan-Meier analyses and Cox proportional hazards regression were conducted using the “Survival” package.
Generation of BCBM specific prognosis scoring model
The TRIPOD statement was applied to generate the new prognosis scoring model (21). Briefly, statistically significant variables associated with patient survival were retained from the multivariate Cox proportional hazards model. Each risk factor was characterized, and the reference value (Wij) was defined as 0 or 1 for categorical factors, and 0 is defined as the base reference value (WiREF). In the molecular subtype, Luminal A was defined as the base reference value. The difference (D) between each risk factor and its base reference value was defined as D=(Wij-WiREF)*βi. The constants B was defined as 0.953. Each risk factor’s point value was calculated as Pointsij=D/B=(Wij-WiREF)*βi/B, and results were rounded to the nearest 1 (Table S1). After the model was established, the estimate of risk (^p) at each corresponding point and time point was calculated as: .
Results
Patient demographics and presentation
Upon retrospective review of electronic medical records for patients surgically treated for BCBM, 214 patients were initially identified. Among them, 27 did not have complete medical records, and 49 were lost follow-up were excluded. The remaining 138 patients met all inclusion and exclusion criteria for the current study and were included. Table 1 summarizes the characteristic of the 138 patients studied. The mean age and BMI for all patients were 54.1±11.5 years old and 23.8±2.9 kg/m2, respectively. The ECOG score and KPS measured patients’ performance status. The previous treatments, including systemic chemotherapy, hormonal therapy, and radiotherapy, were documented. Pathological analysis in terms of molecular type or receptor status of breast cancer was acquired from the bone lesions or previous breast surgery sample. Luminal B (50.6%) from the molecular type and hormone-dependent (76.6%) for receptor status was the most common type. The majority of patients had metastasis located in the spine (60.1%), followed by in the extremities (20.3%) and pelvis (13.9%). All patients were managed, consulted on, and followed up by a multidisciplinary team of radiologists and oncologists. As such, the decision to pursue surgery was made based on the patient’s best interest and clinical judgment by the treating team. All patients had oncologic staging before surgery, and those with significant comorbidities or systemic burden were not offered surgery. The median duration of survival or last follow-up was 34.8 months (95% CI: 26.0–40.7 months) with a range of 0.2–109.1 months. The K-M curve of all patients included in this study was plotted (Figure 1).
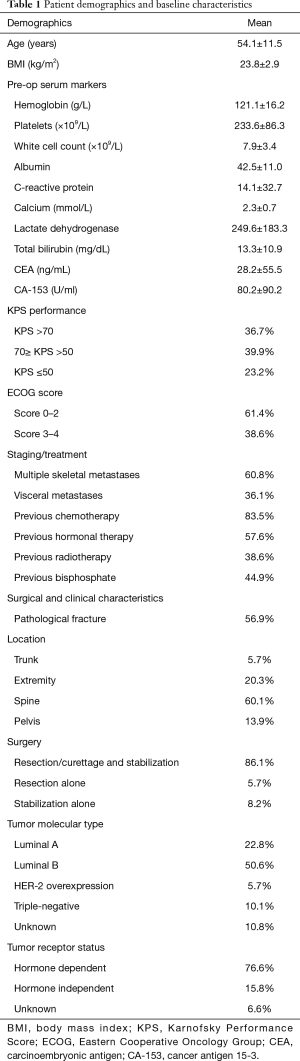
Full table
Factors associated with survival
Firstly, a univariate Cox regression analysis was performed. Older age [≥70 years, hazard ratio (HR) 2.024, 95% CI: 0.993–4.127, P=0.049], higher LDH level (LDH >250 U/L, HR 1.91, 95% CI: 1.130–3.229, P=0.016), poor KPS performance status (KPS <50; HR 2.816, 95% CI: 1.737–4.566, P<0.001), subtype of molecular type (triple-negative subtype, HR 2.09, 95% CI: 1.018–4.289, P=0.045), previous radiotherapy (HR 0.513, 95% CI: 0.320–0.823, P=0.006), presence of pathologic fractures (HR 1.585, 95% CI: 1.001–2.510, P=0.049) and presence of visceral metastases (HR 3.035, 95% CI: 1.893–4.868, P<0.001) were significantly associated with survival (Table 2). Upon multivariate analysis, the following covariates had an independent and statistically significant association with decreased survival: older age (≥70 years old, HR 2.385, 95% CI: 1.099–5.179, P=0.028), poor performance status (KPS <50 HR 2.842, 95% CI: 1.679–4.812, P<0.001), higher LDH level (LDH >250 U/L, HR 2.155, 95% CI: 1.251–3.713, P=0.006), presence of visceral metastases metastasis (HR 2.877, 95% CI: 1.756–4.715, P<0.001), and molecular type (HR 1.312, 95% CI: 1.050–1.641, P=0.017) (Table 3).
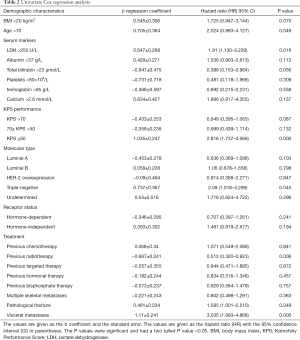
Full table
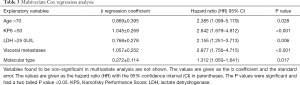
Full table
Overall predictive accuracy of survival by seven scoring systems
Among all patients surgically managed for BCBM, the PathFx model demonstrated the highest accuracy at predicting 3-month and 6-month survival (AUC =0.953 and 0.948, respectively) (Table 4). Other than the PathFx model, Revised katagiri score (AUC =0.904), Janssen nomogram (AUC =0.811), SPRING 13 (AUC =0.717), and SORG Nomogram (AUC =0.7111) also sufficiently accurate at predicting 3-month survival. Revised Katagiri score (AUC =0.7013) and OPTIModel (AUC =0.7418) also provided sufficient accuracy at predicting 6-month survival. PathFx yielded a Brier score of 0.178 at 3-months and 0.272 at 6-months. SSG scale (AUC =0.864) was the most accurate at predicting 12-month survival with a Brier score of 0.275. Additionally, the Janssen nomogram (AUC =0.803), the OPTI model (AUC =0.814), the PathFx model (AUC =0.861), and SORG Nomogram (AUC =0.838) all achieved sufficient predictive accuracy for 12-month survival (Brier score =0.193, 0.260, 0.276, and 0.215 respectively). However, none of these scoring systems were able to achieve satisfactory accuracy for predicting longer than 12-month. In summary, although it failed to provide accurately beyond one year, the PathFx model was the only scoring system to achieve sufficient accuracy at predicting survival within one year after surgery. The revised Katagiri score can also accurately predict survival within 6-month.
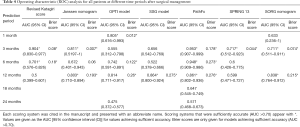
Full table
Generation and examination of BCBM specific prognosis scoring model
Covariates that had an independent and statistically significant association with decreased survival from the multivariate analysis were used to establish a new scoring system. Five variates in the system including older age (age >70), poor KPS performance status (KPS <50), higher LDH level (LDH >250 U/L), presence of visceral metastases, and molecular type of luminal B, triple-negative, or Undetermined. Each category contributes 1 point, with a total score range from 0 to 5 (Table 5). The estimate of the risk of each point at 3-, 6-, 12-, 18-, 24-month was calculated (Table 6). For example, points from 0 to 5 indicate an estimate of risk of 0.09, 0.22, 0.48, 0.81, 0.98, and 0.99 at 24-month. Similarly, a point of 2 indicates an estimate of risk of 0.07, 0.10, 0.22, 0.36 and 0.48 at 3-, 6-, 12-, 18- and 24-month. The K-M curve of patients with different total points showed high distinguish efficiency from each other (log-rank P<0.001) (Figure 2).
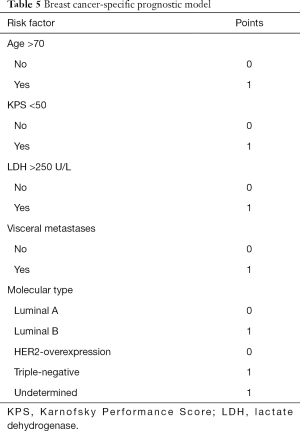
Full table
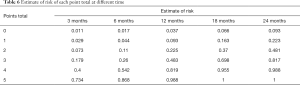
Full table
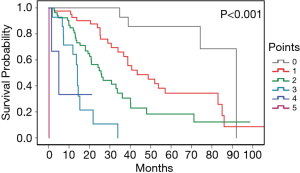
Discussion
This current study validates the seven most relevant prognostic models’ survival prediction accuracy using a non-Western single-center BCBM patient cohort. To our best knowledge, this is one of the most extensive studies comparing the accuracy of different scoring systems and the first study that focuses primarily on BCBM patients.
In our comparison, the PathFx model demonstrated the highest accuracy at predicting 3- and 6-month survival for BCBM patients. It was also the most consistently reliable prognostic system within 12-month. The PathFx model was based on a machine-learning Bayesian belief network, which applies to both axial and appendicular lesions. This model includes both objective and subjective variables. Although only objective elements were applied in our study, the model remained accurate.
Meanwhile, the PathFx model has also been externally validated in different patient populations in European and Asian centers in Japan (22,23). Specifically, a study focused only on femoral metastatic bone disease found a robust prediction accuracy within 6-month (24). As survival of fewer than three months was considered a relative contraindication to surgical management of specific metastatic lesions, the PathFx model can serve as a screening method for this purpose in BCBM patients. Interestingly, the revised Katagiri score also showed accurate prediction with a lower Brier score at 3-month than the PathFx model, indicating that the Revised Katagiri score can also exclude high-risk BCBM patients that may not benefit from surgery.
For the 12-month survival prediction, the SSG Model showed a slightly better predictive accuracy than the PathFx model in our cohort. In the literature, more mixed results were made based on different patient populations. A comparison of nine scoring systems focused on metastatic spine disease found original Tokuhashi score was the most accurate (25). Another similar study focused on femur lesions found OPTI model was better than the PathFx model (24). Although heterogeneity of patient cohorts and study focus may contribute to this inconsistency among studies, it is not surprising to see a decrease in prediction accuracy with the increase of predicted duration.
Our analysis found that none of the seven models included can provide efficient survival prediction beyond 12-month. These seven models were all generated from patients of multiple disease types, including cancers of various prognoses. When specifically applied to breast cancer patients, a cancer type that is considered with relatively favorable prognosis, these models failed to provide an accurate prediction. This finding is discouraging because relative long-term survival prediction is also crucial for surgical planning. Longer life expectancies warrant more-durable reconstruction surgical procedures. Prosthetic reconstruction is preferred in patients with prolonged survival than simple fixation. Thus, highlighting the need to search for new prognostic factors and more specialized approaches to better predict the longer survival in BCBM patients.
The presence of a pathological fracture was found associated with survival only in univariate analysis but not in multivariate analysis in our patient cohort. The SPRING nomogram and PathFx model include preoperative pathological fracture in their assessment, yet the other models do not utilize it for survival estimation (10,11). Studies have reported pathological fracture as a prognostic factor in metastatic cancer patients, however discordant results were also found, and no consensus in BCBM has been achieved yet (4,5,26). Further work and a multi-institutional validation may be required to investigate the prognostic value of pathological fracture. If the pathological fracture was an independent adverse prognostic variable, this would strengthen the argument to lower the threshold of prophylactically surgically managing impending fractures.
Among the serum markers that were included in our study, only LDH showed significance in both univariate and multivariate analyses. LDH is a crucial catalyze in the final step of glycolysis, which is found highly enhanced in cancer status (27). Although elevated plasmatic LDH levels were found in various malignancies, few prognostic studies put it into consideration, and were only included by the revised Katagiri score model (27). In fact, the LDH level has been correlated strongly with survival, especially in BCBM patients across different cohorts (28-30). A meta-analysis that included more than six thousand patients further confirmed LDH as a prognostic factor in breast cancer patients (31). Additionally, persistent elevated LDH levels and LDH low-to-high variation was strongly correlated with worse chemo-response and progression-free survival (32,33). These results corroborate our finding that LDH is a prognostic factor in BCBM patients and contribute to prognostic models. Further meta-analysis studies are still needed to clarify whether the prognostic role of LDH only exists in breast cancer rather than other cancer types. Another issue about LDH is that it can be easily affected by systemic treatment or other comorbidities. Multiple testing or a trend of LDH should be considered instead of a single-time result when applied to the prognostic analysis.
An increasing number of studies have shown that breast cancer molecular subtypes, the classification into either a luminal A, luminal B, HER-2 overexpression, or triple-negative tumors, are biologically distinct, respond differently to adjuvant therapy, and have different outcomes (3,34-37). Additional studies suggest that tumor biology and molecular heterogeneity within breast cancer subtypes, rather than therapy choice, determined the survival trends (13,14). Consistent with that, our study revealed that the survival of BCBM patients in our cohort differs by subtype, suggesting that subtype classification is clinically useful and will help determine estimated survival. In literature, luminal A tumors showed the best, and triple-negative tumors presented the poorest outcome across studies and ethnicities (3,34-36), while those with missing receptor status tend to have worse prognostic features (38). Besides, positive HER-2 expression is related to better survival regardless of hormone receptor status (38,39). Understanding the biological nature and prognosis of molecular subtypes will benefit future prognostic analysis and improve prediction accuracy. It also explains the moderate accuracy in survival prediction models that consider all types of breast cancer as a whole.
The prognostic model proposed in this study after considering the molecular subtypes. Besides, this model is an easy-to-use scoring system with only five variables and a range of 0 to 5 points in total. This model overcomes the relative long-term survival prediction gap for BMBC patients. Although further studies are still needed to validate this model, it provides help with surgical planning. Take extremity metastasis as an example, since resection and prosthetic reconstruction is preferred in patients with more prolonged survival (24,26), patients who scored 0–2 points by our model could be potential candidates for prosthetic reconstruction rather than nailing alone if surgical management is deemed necessary. However, we did notice that our prediction model lose its distinguishability after 30 months for patients rated within 0-2 points. The relatively small total sample size in this study and fewer survivors after 80 months may explain this. Additionally, the prediction accuracy also decreases when applied to a more extended survival prediction due to the increase of uncontrollable interfering factors. More comprehensive analysis is needed when applying our proposed model for more extended survival prediction. Fortunately, this imperfection did not affect clinical usage and surgical decision-making.
There are several limitations in this study. Possible selection bias may present in this study inherently associated with retrospective studies, the sample size is also relatively small, in which 66 out of 204 patients were excluded in this study due to a lack of crucial information or loss of follow-up. Patients included in this study were not randomly allocated to treatment. Although each patient’s treatment plan was made by multidiscipline consultation, the surgical team’s surgical treatment decisions were not based on standardized protocols. Further, applying the previous prognostic models to a potentially homogenous breast cancer patient group may introduce bias to the analysis and render the accuracy. Additionally, the newly developed prognostic model was based on data from a single institution. As such, further prospective multi-institutional studies are appropriate to replicate and confirm the findings presented here.
Conclusions
Accurate preoperative estimated patient survival is paramount to an informed operative plan and treatment decision-making for BCBM patients. The PathFx model demonstrated superior accuracy at predicting short-term survival after surgery than other models for Asian BCBM patients. Although multiple prognostic models are available, none of them provide sufficient prediction beyond one year. A better long-term estimation is needed for breast cancer patients, which are generally considered with relatively more prolonged survival as compared to other cancer types. Since cancer biology plays a dominant role in survival, identify cancer-type specific prognostic variables will improve survival prediction accuracy. Our proposal for an easy-to-use scoring system fills the gap for long-term survival predictions for BCBM patients after adding cancer-specific prognostic factors. Although further validation is needed, the Modification in this present study can improve clinical decision-making in patients with metastatic breast cancer and offer a more individualized tool for informing patients.
Acknowledgments
We thank Dr. Siyao Liu and Ms. Yanchun She for their work on patients’ enrollment and follow-up.
Funding: None.
Footnote
Provenance and Peer Review: This article was commissioned by the editorial office, Annals of Joint for the series “Bone Metastasis”. The article has undergone external peer review.
Reporting Checklist: The authors have completed the STROBE reporting checklist. Available at http://dx.doi.org/10.21037/aoj-20-120
Data Sharing Statement: Available at http://dx.doi.org/10.21037/aoj-20-120
Conflicts of Interest: All authors have completed the ICMJE uniform disclosure form (available at http://dx.doi.org/10.21037/aoj-20-120). The series “Bone Metastasis” was commissioned by the editorial office without any funding or sponsorship. RY served as the unpaid Guest Editor of the series. The authors have no other conflicts of interest to declare.
Ethical Statement: The authors are accountable for all aspects of the work in ensuring that questions related to the accuracy or integrity of any part of the work are appropriately investigated and resolved. This research was conducted in accordance with the Declaration of Helsinki (as revised in 2013). The study was approved by the Ethics Committee of Peking University People’s Hospital. Informed consent was not required for this retrospective, non-interventional study, which was considered minimal risk.
Open Access Statement: This is an Open Access article distributed in accordance with the Creative Commons Attribution-NonCommercial-NoDerivs 4.0 International License (CC BY-NC-ND 4.0), which permits the non-commercial replication and distribution of the article with the strict proviso that no changes or edits are made and the original work is properly cited (including links to both the formal publication through the relevant DOI and the license). See: https://creativecommons.org/licenses/by-nc-nd/4.0/.
References
- Siegel RL, Miller KD, Jemal A. Cancer statistics, 2020. CA Cancer J Clin 2020;70:7-30. [Crossref] [PubMed]
- Chen W, Sun K, Zheng R, et al. Cancer incidence and mortality in China, 2014. Chin J Cancer Res 2018;30:1-12. [Crossref] [PubMed]
- Wang R, Zhu Y, Liu X, et al. The Clinicopathological features and survival outcomes of patients with different metastatic sites in stage IV breast cancer. BMC Cancer 2019;19:1091. [Crossref] [PubMed]
- Coleman RE. Clinical features of metastatic bone disease and risk of skeletal morbidity. Clin Cancer Res 2006;12:6243s-9s. [Crossref] [PubMed]
- Saad F, Lipton A, Cook R, et al. Pathologic fractures correlate with reduced survival in patients with malignant bone disease. Cancer 2007;110:1860-7. [Crossref] [PubMed]
- Katagiri H, Okada R, Takagi T, et al. New prognostic factors and scoring system for patients with skeletal metastasis. Cancer Med 2014;3:1359-67. [Crossref] [PubMed]
- Janssen SJ, van der Heijden AS, van Dijke M, et al. 2015 Marshall Urist Young Investigator Award: Prognostication in Patients With Long Bone Metastases: Does a Boosting Algorithm Improve Survival Estimates? Clin Orthop Relat Res 2015;473:3112-21. [Crossref] [PubMed]
- Willeumier JJ, van der Linden YM, van der Wal C, et al. An Easy-to-Use Prognostic Model for Survival Estimation for Patients with Symptomatic Long Bone Metastases. J Bone Joint Surg Am 2018;100:196-204. [Crossref] [PubMed]
- Ratasvuori M, Wedin R, Keller J, et al. Insight opinion to surgically treated metastatic bone disease: Scandinavian Sarcoma Group Skeletal Metastasis Registry report of 1195 operated skeletal metastasis. Surg Oncol 2013;22:132-8. [Crossref] [PubMed]
- Forsberg JA, Eberhardt J, Boland PJ, et al. Estimating survival in patients with operable skeletal metastases: an application of a bayesian belief network. PLoS One 2011;6:e19956 [Crossref] [PubMed]
- Sørensen MS, Gerds TA, Hindsø K, et al. External Validation and Optimization of the SPRING Model for Prediction of Survival After Surgical Treatment of Bone Metastases of the Extremities. Clin Orthop Relat Res 2018;476:1591-9. [Crossref] [PubMed]
- van der Linden YM, Dijkstra SP, Vonk EJ, et al. Prediction of survival in patients with metastases in the spinal column: results based on a randomized trial of radiotherapy. Cancer 2005;103:320-8. [Crossref] [PubMed]
- Ambs S. Prognostic significance of subtype classification for short- and long-term survival in breast cancer: survival time holds the key. PLoS Med 2010;7:e1000281 [Crossref] [PubMed]
- Blows FM, Driver KE, Schmidt MK, et al. Subtyping of breast cancer by immunohistochemistry to investigate a relationship between subtype and short and long term survival: a collaborative analysis of data for 10,159 cases from 12 studies. PLoS Med 2010;7:e1000279 [Crossref] [PubMed]
- Perou CM, Sørlie T, Eisen MB, et al. Molecular portraits of human breast tumours. Nature 2000;406:747-52. [Crossref] [PubMed]
- Sørlie T, Perou CM, Tibshirani R, et al. Gene expression patterns of breast carcinomas distinguish tumor subclasses with clinical implications. Proc Natl Acad Sci U S A 2001;98:10869-74. [Crossref] [PubMed]
- Paulino Pereira NR, McLaughlin L, Janssen SJ, et al. The SORG nomogram accurately predicts 3- and 12-months survival for operable spine metastatic disease: External validation. J Surg Oncol 2017;115:1019-27. [Crossref] [PubMed]
- Forsberg JA, Wedin R, Bauer HC, et al. External validation of the Bayesian Estimated Tools for Survival (BETS) models in patients with surgically treated skeletal metastases. BMC Cancer 2012;12:493. [Crossref] [PubMed]
- Harrell FE Jr, Lee KL, Mark DB. Multivariable prognostic models: issues in developing models, evaluating assumptions and adequacy, and measuring and reducing errors. Stat Med 1996;15:361-87. [Crossref] [PubMed]
- DeLong ER, DeLong DM, Clarke-Pearson DL. Comparing the areas under two or more correlated receiver operating characteristic curves: a nonparametric approach. Biometrics 1988;44:837-45. [Crossref] [PubMed]
- Collins GS, Reitsma JB, Altman DG, et al. Transparent reporting of a multivariable prediction model for individual prognosis or diagnosis (TRIPOD): the TRIPOD statement. Bmj 2015;350:g7594. [Crossref] [PubMed]
- Ogura K, Gokita T, Shinoda Y, et al. Can A Multivariate Model for Survival Estimation in Skeletal Metastases (PATHFx) Be Externally Validated Using Japanese Patients? Clin Orthop Relat Res 2017;475:2263-70. [Crossref] [PubMed]
- Piccioli A, Spinelli MS, Forsberg JA, et al. How do we estimate survival? External validation of a tool for survival estimation in patients with metastatic bone disease-decision analysis and comparison of three international patient populations. BMC Cancer 2015;15:424. [Crossref] [PubMed]
- Meares C, Badran A, Dewar D. Prediction of survival after surgical management of femoral metastatic bone disease - A comparison of prognostic models. J Bone Oncol 2019;15:100225 [Crossref] [PubMed]
- Ahmed AK, Goodwin CR, Heravi A, et al. Predicting survival for metastatic spine disease: a comparison of nine scoring systems. Spine J 2018;18:1804-14. [Crossref] [PubMed]
- Mavrogenis AF, Pala E, Romagnoli C, et al. Survival analysis of patients with femoral metastases. J Surg Oncol 2012;105:135-41. [Crossref] [PubMed]
- Wulaningsih W, Holmberg L, Garmo H, et al. Serum lactate dehydrogenase and survival following cancer diagnosis. Br J Cancer 2015;113:1389-96. [Crossref] [PubMed]
- Brown JE, Cook RJ, Lipton A, et al. Serum lactate dehydrogenase is prognostic for survival in patients with bone metastases from breast cancer: a retrospective analysis in bisphosphonate-treated patients. Clin Cancer Res 2012;18:6348-55. [Crossref] [PubMed]
- Chen B, Dai D, Tang H, et al. Pre-treatment serum alkaline phosphatase and lactate dehydrogenase as prognostic factors in triple negative breast cancer. J Cancer 2016;7:2309-16. [Crossref] [PubMed]
- Agrawal A, Gandhe MB, Gupta D, et al. Preliminary Study on Serum Lactate Dehydrogenase (LDH)-Prognostic Biomarker in Carcinoma Breast. J Clin Diagn Res 2016;10:BC06-8. [Crossref] [PubMed]
- Liu D, Wang D, Wu C, et al. Prognostic significance of serum lactate dehydrogenase in patients with breast cancer: a meta-analysis. Cancer Manag Res 2019;11:3611-9. [Crossref] [PubMed]
- Pelizzari G, Basile D, Zago S, et al. Lactate Dehydrogenase (LDH) Response to First-Line Treatment Predicts Survival in Metastatic Breast Cancer: First Clues for A Cost-Effective and Dynamic Biomarker. Cancers (Basel) 2019;11:1243. [Crossref] [PubMed]
- Dennison JB, Molina JR, Mitra S, et al. Lactate dehydrogenase B: a metabolic marker of response to neoadjuvant chemotherapy in breast cancer. Clin Cancer Res 2013;19:3703-13. [Crossref] [PubMed]
- Hennigs A, Riedel F, Gondos A, et al. Prognosis of breast cancer molecular subtypes in routine clinical care: A large prospective cohort study. BMC Cancer 2016;16:734. [Crossref] [PubMed]
- Fallahpour S, Navaneelan T, De P, et al. Breast cancer survival by molecular subtype: a population-based analysis of cancer registry data. CMAJ Open 2017;5:E734-9. [Crossref] [PubMed]
- Parkes A, Clifton K, Al-Awadhi A, et al. Characterization of bone only metastasis patients with respect to tumor subtypes. NPJ Breast Cancer 2018;4:2. [Crossref] [PubMed]
- Bollen L, Wibmer C, Wang M, et al. Molecular phenotype is associated with survival in breast cancer patients with spinal bone metastases. Clin Exp Metastasis 2015;32:1-5. [Crossref] [PubMed]
- Howlader N, Cronin KA, Kurian AW, et al. Differences in Breast Cancer Survival by Molecular Subtypes in the United States. Cancer Epidemiol Biomarkers Prev 2018;27:619-26. [Crossref] [PubMed]
- Gong Y, Liu YR, Ji P, et al. Impact of molecular subtypes on metastatic breast cancer patients: a SEER population-based study. Sci Rep 2017;7:45411. [Crossref] [PubMed]
Cite this article as: Du W, Wang J, Xu J, Zhao Z, Liu S, Yang L, Yang R, Wang S, Guo W. Comparison and modification of survival predicting system for breast cancer patients with bone metastases. Ann Joint 2021;6:28.